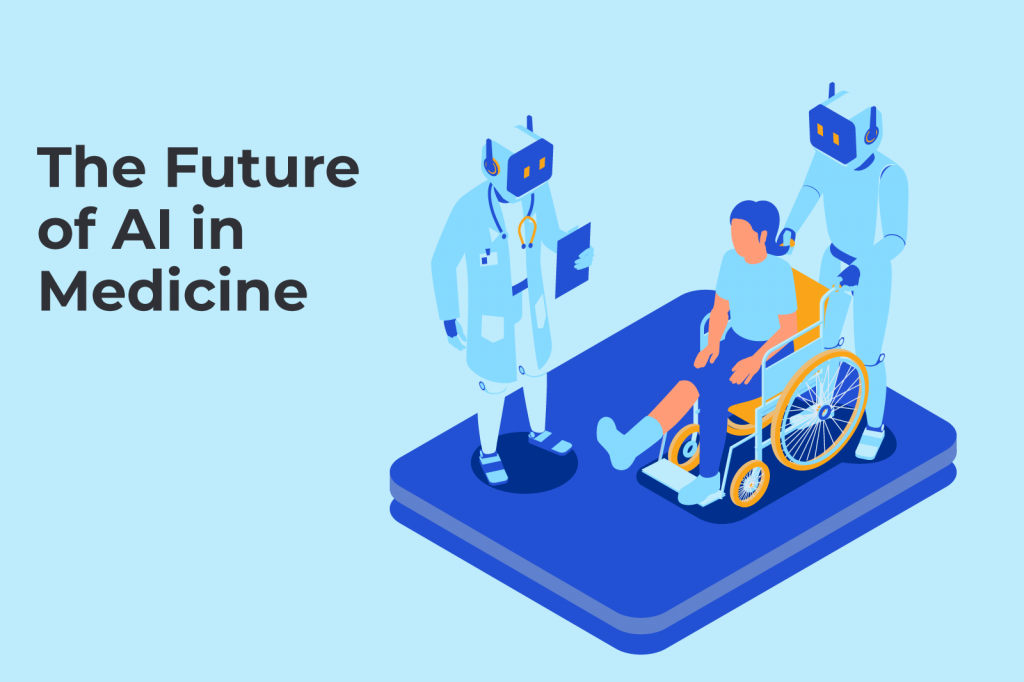
The Future of AI in Medicine
Many perceive AI as not just a thing of the present but, more importantly, an essential part of the future. As the field continues to make technological leaps, its importance can't be denied even in fields that require a great degree of specialization, such as the medical one. In this article, we try to make a small picture of the moment—of where AI currently stands in the medical field and explore some of its potential evolution and challenges that it will need to face in the future.
How is AI changing the clinical decision-making process?
In Medicine, Artificial intelligence is usually used to help the clinical decision-making process based on insights extracted from past data. Before AI emerged, clinicians used statistical methods to identify patterns and predict outcomes. While mathematical equations are still being broadly used, AI can bring more to the table. Firstly, it's the "connecting the dots" sort to speak ability of neural networks to discover complex relationships that can't be simply attributed to an equation. Like a human brain, neural networks can pose serious problem-solving skills, thus making them more adaptable and efficient.
But medical professionals can do that by themselves, right?
Yes, but clinicians can only assess a limited volume of data in a specific time frame. At the same time, machine learning systems can work with millions of data points simultaneously in a very short time. Also, ML (machine learning applications) can improve incrementally over time as they are exposed to more cases and data.
Where can AI truly shine in Medicine?
It's probably no surprise, but AI really manages to shine when handling well-defined and/or repetitive tasks. According to a study, AI managed to have superior precision than dermatologists in classifying biopsy-validated skin lesions based on photographs. Even though this sounds impressive, we must consider these results are obtained based on the task typology - they have precise inputs and outputs - the input is a photo, and the output is a binary classification: benign or malignant. Still, this example encompasses how AI can improve the accuracy and speed of medical professionals in specific tasks, but does this mean they might replace them one day?
Another use case of an AI development trend in medicine is one of our custom in-house AI chatbots that can manage, categorize, and interpret medical data and specialized equipment manuals. This secure, on-premise approach can provide medical professionals with fast and precise replies to their questions - these could include results from certain studies, medical or internal procedures, equipment troubleshooting and maintenance, and much more. If you want to learn more about our custom AI chatbots for the medical field contact us here.
Another context where AI can make a huge difference is where human resources are scarce; many countries and/or regions worldwide have a high deficit of medical staff and resources. In such circumstances, specific AI applications can have an even more significant perceived impact as they can save precious time on an already limited staff list. According to another study, radiographs used AI to interpret massive amounts of scans. AI correctly diagnosed pulmonary TB with a sensitivity of 95% and a specificity of 100%.
Currently, there is much more to a medical professional than just their knowledge and skill. There's also empathy and compassion, traits that AI systems don't possess and are vital for building a relationship based on trust between the patient and their doctor. Even though AI manages to greatly overpass a doctor in the sheer number of data points it can process in a short time frame, that doesn't mean it will replace them. Still, it will most likely become one of the most critical tools in a medical professional arsenal. Another essential point to consider is the emotional/psychological impact that an authentic, human interaction has over a patient and how AI is still seen with mistrust - this is critical as the mass perception of AI will most likely define how far it will integrate into the medical field - will it take the storefront or remain a background but valuable tool?
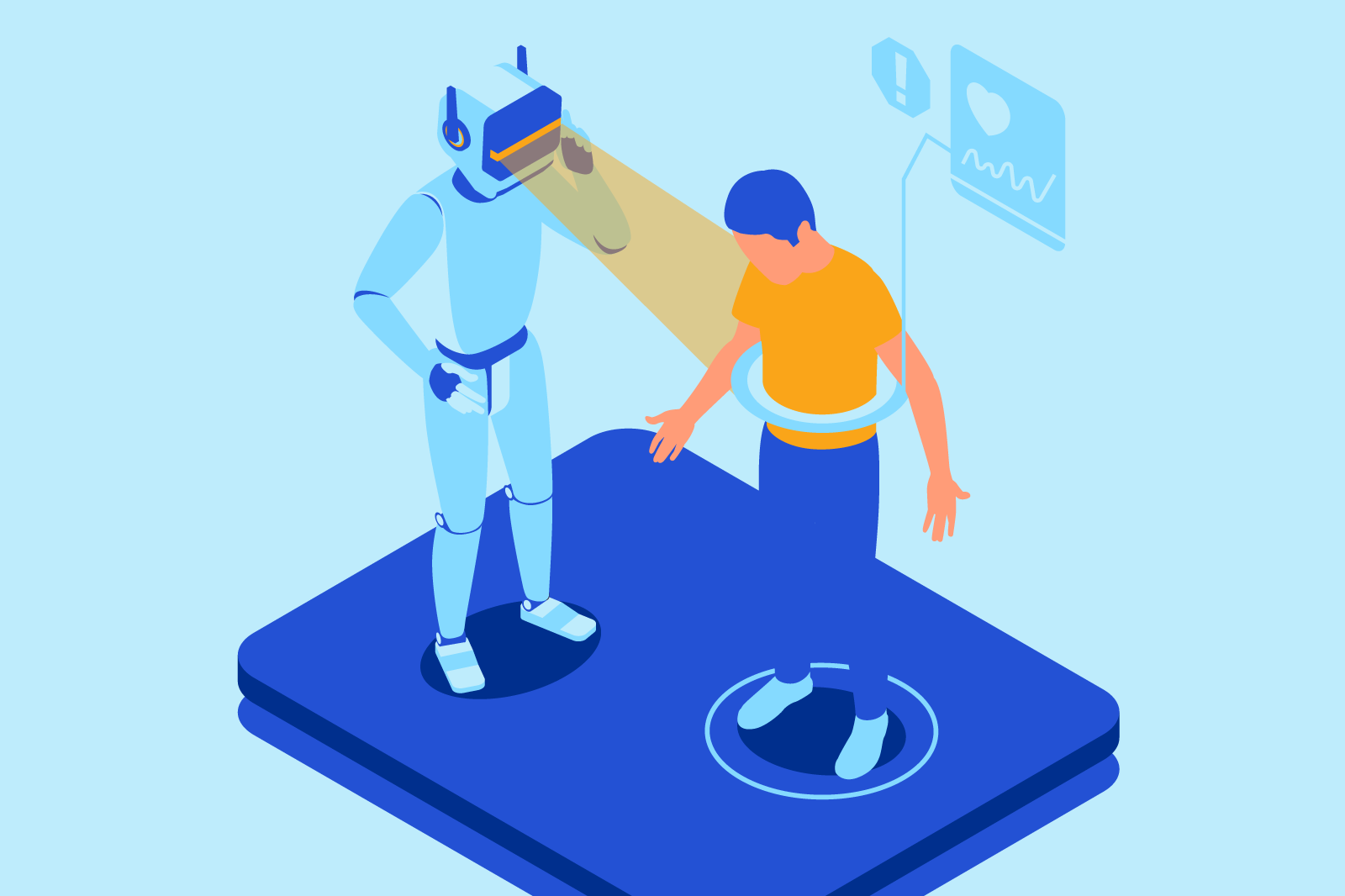
AI Trends in Healthcare - Our Digital Medical Footprint.
There are things that require a predefined infrastructure to ensure AI can reach its potential in the medical world. As more and more countries and medical systems implement individual digital medical footprints and connect all data sources into one centralized system, AI-based applications will become more efficient and better at predicting. More than that, AI can unlock personalized treatment due to its capacity to manage and interpret millions of data points and immense quantities of data; with their use, medical professionals will be able to ditch the "one-size-fits-all" treatments and implement highly personalized plans.
Preventive Medicine is also a field in which AI can become essential for the same reasons. They can identify patterns and assess health risks if they have access to our digital medical footprint. Furthermore, based on the risk assessment, they could assign lower waiting times for higher-risk patients in a truly connected infrastructure.
What are AI's limitations in its adoption into the medical field?
One of the essential limitations of AI and ML models is their requirement for massive amounts of data and a certain level of quality. Nonetheless, the data used to train this model to make predictions must be formatted appropriately (which requires skilled data engineers) and, more importantly, stable over a certain amount of time. To try to display this, imagine a process that has stable outcomes. For example, on a production line, the output can be predicted precisely based on multiple factors, from machinery downtime to personnel efficiency. These conditions are often stable, and the conditions can be easily changed and implemented in a system when, for example, equipment is changed to a better one, thus resulting in a better output. AI can struggle when data is drastically inconsistent or its patterns are often changed.
In a real-life example, in 2008, Google tried to predict the seasonal prevalence of influenza. However, its AI model used only the search terms entered into the search engine, which are highly influenced by changing search habits and trends. Due to its lack of accuracy, the AI model was discontinued.
Ready to write the future of Medicine with AI?
Our dedicated AI team can implement complex solutions and leverage AI capabilities to transform your processes, save time, and improve prediction. From AI chatbots to NLP systems, our services are always tailored to your specific needs, keeping quality and cost-efficiency in mind.
Register on our AI test platform to discover some of our custom AI capabilities, and contact us for a personalized demo and quote.
Article written by Iulia Filimon, NLP Engineer
Iulia designs and develops algorithms and models that enable computers to understand, interpret, and generate human language. She participates in building the first innovative AI company's solutions and exposing them to clients.